Business IntelligenceData Warehouse vs Data Lake: Why You Don't Have To Choose
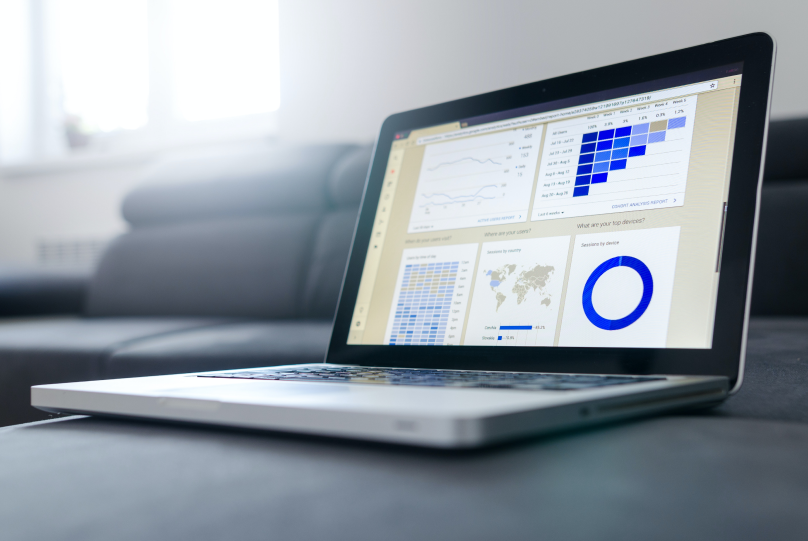
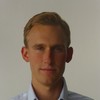
Andrzej Gut
BI & Data Science Team Leader

Stanislav Naborshchikov
Solutions Specialist
Table of contents
Share the article
Introduction
In today's data-driven world, modern businesses face the challenge of harnessing the vast and relentless influx of data from multiple sources. Organizations grapple with large amounts of information streaming in from various channels as the digital landscape expands. In response to this data deluge, two prominent solutions have emerged as saviors for data management: Data warehouse vs Data Lake. Each approach offers distinct advantages and has dedicated use cases, leading to an ongoing debate over which one reigns supreme. However, beneath the seemingly binary choice lies a more nuanced reality - Data Lake and Data Warehouse are not rivals; they are formidable allies. In many cases, their successful integration can amplify an organization's analytical capabilities and provide a comprehensive data management strategy.
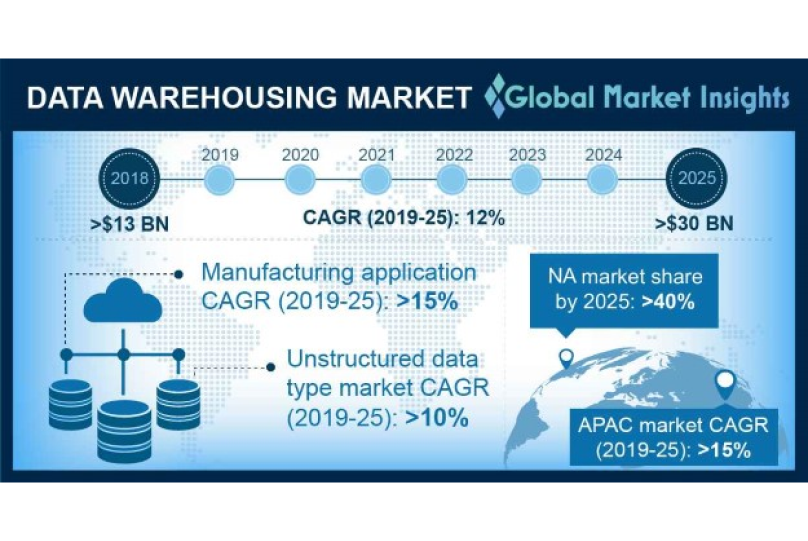
Source: https://www.gminsights.com/industry-analysis/data-warehousing-market
Polaris reports that demand for DWaaS (Data warehouse as a service) is poised for significant growth due to the escalating volume of structured and unstructured data generated across diverse industries such as BFSI, government, manufacturing, and more. The advent of cutting-edge technologies, including AI (artificial intelligence), cloud computing, and IoT (internet of things), presents immense growth opportunities for these services and the growth of the popularity of data warehouses of data lake-related solutions. Furthermore, the increasing adoption of AI and machine learning in computing solutions is expected to propel the expansion of the data warehouse-as-a-service market.
Key market players' recent developments have fueled the demand for these services. For example, in March 2020, Google launched the Google AI cloud platform, providing a platform to design and deploy machine learning product pipelines. Additionally, strategic mergers and partnerships in the competitive landscape are anticipated to boost industry growth. A notable example is the partnership between IBIOS IT and IBM in September 2019 to deliver POWER9 solutions.
Furthermore, in May 2021, WPP joined forces with Microsoft to transform content production with the Cloud Studio. These collaborations demonstrate the increasing focus on leveraging innovative technologies to enhance data warehousing capabilities and drive industry expansion.
This article delves into Data warehouse vs Data Lake, exploring their unique strengths and uncovering the untapped potential when these two robust systems join harmonious forces. With Data Lakes providing the ability to ingest and store large amounts of raw and semi-structured data, organizations can effectively handle diverse data sources. Meanwhile, Data Warehouses offer robust data quality and relational database capabilities, ensuring data integrity and reliability for sophisticated data analysis. Moreover, cloud-based platforms like Microsoft Azure and Amazon S3 have further enhanced the integration of Data Lakes and Data Warehouses, facilitating seamless data movement and storage and paving the way for organizations to thrive in the data-driven landscape.
What is data warehouse?
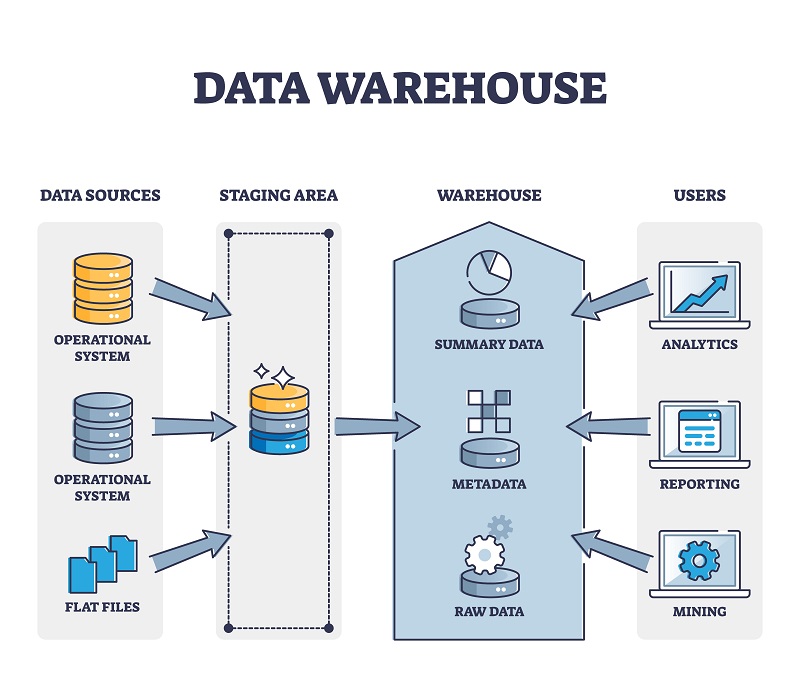
Data warehouse definition
A data warehouse is a centralized repository that stores, integrates, and manages large volumes of data from various sources within an organization. It's designed to support business intelligence (BI) activities and decision-making processes by providing a structured and optimized environment for data analysis, reporting, and querying. Data warehouses are typically used for big data processing and consolidating data from disparate sources into a single location, making extracting meaningful insights and trends more accessible.
Key features of a data warehouse include:
Subject-Oriented
Data warehouses are organized around specific subjects or business areas, such as sales, marketing, finance, or operations. This organization allows users to focus on a particular aspect of the business and analyze data related to that area.
Integrated Data
Data warehouses integrate data from various sources, which may include operational databases, external systems, spreadsheets, and more. This integration ensures that all relevant data is available for analysis without the need to query multiple systems separately.
Time-Variant
Data warehouses store historical data over time, allowing users to analyze trends and changes in the data. This time-variant aspect is crucial for conducting trend analysis, identifying patterns, and making informed historical comparisons.
Non-Volatile
Data in a data warehouse is considered non-volatile, meaning that once data is loaded into the warehouse, it is not frequently updated, deleted, or altered. Instead, new data is appended, and historical data is retained for analysis.
Optimized for Query Performance
Data warehouses are designed for efficient querying and reporting. They use techniques like indexing, partitioning, and data denormalization to improve query performance, enabling users to retrieve insights quickly, even from large datasets.
Aggregated Data
Data warehouses often contain pre-aggregated data to speed up analytical queries. Aggregations like sums, averages, counts, and other computations are pre-calculated and stored, reducing the need for complex calculations during query execution.
Support for Complex Queries
Data warehouses support complex SQL queries that involve joining multiple tables, filtering data, and performing calculations. This flexibility allows users to perform in-depth analysis and generate custom reports.
Data Quality and Consistency
Data warehouses typically include mechanisms to ensure data quality and consistency. This involves data cleansing, transformation, and validation processes to maintain accuracy and reliability.
Decision Support
Data warehouses are primarily built to support decision-making processes. Business analysts, executives, and other users can use the data warehouse to gain insights into the organization's performance, identify opportunities, and make informed decisions.
Separation from Operational Systems
Data warehouses are separate from the operational databases that support day-to-day business activities. This separation prevents analytical queries from impacting the performance of operating systems.
Overall, data warehouses play a crucial role in turning raw data into valuable insights, enabling businesses to make informed decisions based on comprehensive and well-organized data.
Running a traditional data warehouse offers several advantages and comes with some challenges as well. Here are the pros and cons of operating a data warehouse:
Data warehouse benefits
Data warehouse benefits encompass a range of advantages that support and enhance organizational operations. A well-maintained data warehouse acts as a centralized source of reliable and integrated data, which is crucial for data-driven decision-making across an organization. It facilitates deep analysis of both historical and current data, helping to uncover trends, patterns, and correlations that lead to valuable business insights and strategic planning.
In terms of performance, data warehouses are optimized for querying and reporting, which results in faster query performance and an improved user experience when handling large datasets. This optimization is coupled with the advantage of data consistency. By consolidating data from various sources, data warehouses help in maintaining consistent and standardized data, thereby reducing the chances of discrepancies and errors.
Scalability is another key benefit. Many modern data warehouses offer scalability options, enabling organizations to expand their storage and processing capacity in line with growing data requirements. This flexibility extends to querying capabilities as well. Data warehouses support complex SQL queries, empowering analysts to explore data from multiple angles, apply various filters, and perform detailed aggregations.
For organizations looking to engage in advanced analytics, data warehouses provide a solid foundation. This enables the pursuit of activities like predictive modeling, machine learning, and data mining, which can offer a competitive edge. Additionally, centralizing data in a data warehouse can significantly aid in adhering to data governance and compliance regulations. Access to and usage of data can be monitored and controlled more effectively, ensuring regulatory compliance and enhancing overall data management.
Cons of data warehouse
Data warehouses, while beneficial for improved data analysis and decision-making, come with their own set of challenges that organizations need to carefully consider. The implementation of a data warehouse can be complex and time-consuming, requiring significant efforts in data integration, transformation, and ongoing monitoring. This process often involves high initial costs, including expenses for hardware, software, and hiring skilled personnel, which can be a substantial investment, especially for smaller organizations.
One of the primary challenges in setting up a data warehouse is integrating data from various sources. This task can be difficult due to differences in data formats, structures, and quality, necessitating extensive data cleansing and transformation efforts. Additionally, centralizing data in one location raises significant data security concerns, making it crucial to implement robust security measures to protect sensitive information.
The maintenance of a data warehouse is another factor that demands attention. Regular maintenance tasks like updates, backups, performance tuning, and monitoring can consume considerable time and resources. Furthermore, the issue of data freshness arises, as there might be delays between the generation of data in operational systems and its availability in the warehouse.
Organizations also face the risk of vendor lock-in when choosing proprietary data warehouse solutions, which can limit flexibility and potentially increase costs. Moreover, data warehouses require a demand for skilled personnel, such as data engineers, database administrators, and analysts, to manage and operate them effectively.
Scalability, although offered by many data warehouses, can present its own challenges. Expanding the system's capacity might involve additional costs and technical complexities. Lastly, getting business users and teams to adopt and effectively use the data warehouse tools and insights can sometimes be challenging, necessitating training and change management efforts.
In summary, while data warehouses offer substantial benefits, they also pose various challenges that require careful planning and management to ensure the effective use of data warehouse initiatives.
Do you need support with your business intelligence architecture and process?
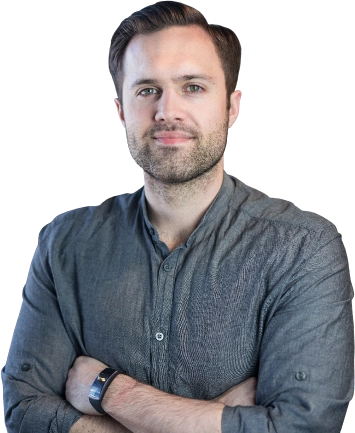
Or contact us:
Data warehouse providers
As we know what data warehouse is, what features it has, and what are advantages and disadvantages of data warehouse let's explore what companies provide data warehouse as a service:
Amazon Redshifts
Amazon Redshift is a game-changer for tens of thousands of customers seeking a fast and fully managed cloud data warehouse service.
It is engineered to manage datasets of petabyte scale, and this cutting-edge solution simplifies and cost-effectively enables efficient data analysis using existing business intelligence tools. With optimization tailored for datasets ranging from a few hundred gigabytes to a petabyte or more, Amazon Redshift proves to be a scalable and budget-friendly option.
Costing less than $1,000 per terabyte per year, it presents a tenth of the expense compared to most traditional data warehousing solutions. Businesses of all sizes can leverage Amazon Redshift to unlock the potential of their data, conducting in-depth analysis with ease. The platform's seamless integration with existing tools ensures a smooth transition and empowers teams to make data-driven decisions without costly overhauls.
As a fully managed service, Amazon Redshift reduces administrative burdens, freeing up valuable resources and time for businesses to focus on deriving insights and enhancing productivity. With its exceptional performance, cost-efficiency, and scalability, Amazon Redshift sets a new standard for data warehousing, revolutionizing the way businesses analyze and utilize their data.
Google Cloud BigQuery
BigQuery stands as a revolutionary serverless, multicloud data warehouse designed to streamline data management and accelerate the generation of valuable business insights. As a pivotal component of Google's data cloud, BigQuery empowers businesses to focus on their analytics without getting bogged down by complex data processing.
With BigQuery, data integration becomes a breeze, enabling seamless collaboration across various data sources. The platform's serverless architecture eliminates the need for manual infrastructure management, allowing businesses to optimize costs and allocate resources more efficiently.
Scalability is at the heart of BigQuery's capabilities, ensuring that analytics can grow alongside your business needs. Additionally, the platform emphasizes data security, providing peace of mind when dealing with sensitive information.
One of BigQuery's key strengths lies in its built-in business intelligence, offering robust data visualization tools for creating rich data experiences. This integration fosters a data-driven culture within organizations, enabling teams to uncover valuable insights and make informed decisions.
Furthermore, BigQuery facilitates machine learning initiatives through a simple SQL interface, streamlining the process of training and deploying ML models. This integration empowers businesses to leverage data for predictive analytics, further enhancing the organization's operations.
Snowflake
Snowflake is a pioneering platform that powers the Data Cloud, a global network empowering thousands of organizations to mobilize data with unparalleled scale, concurrency, and performance. Within the Data Cloud, businesses can unify their disparate data sources, seamlessly discover and securely share governed data, and execute diverse analytic workloads. With Snowflake, data silos become a thing of the past as organizations gain access to a single, unified experience across multiple public clouds.
This flexibility enables businesses to seamlessly integrate data from various sources and locations, empowering them to make data-driven decisions with confidence. Snowflake's platform serves as the engine that drives and provides access to the Data Cloud, supporting various data-related initiatives such as data warehousing, data lake system, data engineering, data science, data application development, and data sharing. By offering a comprehensive suite of solutions, Snowflake enables businesses to unlock the true potential of their data and drive innovation across all aspects of their operations.
When should I use data warehouse?
A data warehouse is best suited for scenarios where you need to support business intelligence (BI) and decision-making processes that involve structured data analysis, reporting, and historical trend analysis. Here are some situations in which using a data warehouse is appropriate:
Structured Data Analysis:
When your data is well-structured and comes from various operational systems, a data warehouse can integrate and organize this data in a consistent manner, making it easier to analyze.
Complex Queries:
If your organization requires complex SQL queries involving multiple tables, aggregations, and calculations, a data warehouse's optimized query performance can significantly speed up the analysis process.
Historical Analysis:
If you need to analyze historical data trends, patterns, and changes over time, a data warehouse's capability to store and manage historical data makes it a suitable choice.
Business Reporting:
When you need to generate standard and ad hoc reports for business users, a data warehouse provides a structured environment to create consistent and accurate information.
Data Integration:
If your data is scattered across various databases, systems, or departments, a data warehouse can centralize and integrate the data, reducing the need to query multiple sources separately.
Data Consistency:
When data consistency and accuracy are critical for decision-making, a data warehouse can enforce data quality measures and standardized formats.
Performance Optimization:
If your operational systems are not optimized for analytical queries, using a data warehouse can offload analytical processing, ensuring that operating systems remain responsive.
Regulatory Compliance:
When you need to adhere to data governance and compliance regulations, a data warehouse's ability to control data access and maintain audit trails can be beneficial.
Large Data Volumes:
If you're dealing with large volumes of data that need to be processed and analyzed efficiently, a data warehouse's optimization for query performance can be advantageous.
Structured Business Processes:
If your organization follows well-defined business processes and reporting requirements, a data warehouse can support these processes by providing a centralized source of accurate and consistent data.
Data Transformation and Enrichment:
When data needs to be transformed, cleaned, and enriched before analysis, a data warehouse can facilitate these processes to ensure high-quality data.
Structured Analytics:
If your organization mainly focuses on structured analytics, such as creating dashboards, scorecards, and standard KPI reports, a data warehouse can provide a solid foundation.
In essence, use a data warehouse when you need a structured, optimized environment for querying and reporting on well-structured data to support business intelligence initiatives and informed decision-making. It's particularly beneficial for scenarios involving historical analysis, complex queries, and reporting needs that require accurate and consistent data.
What is data lake?
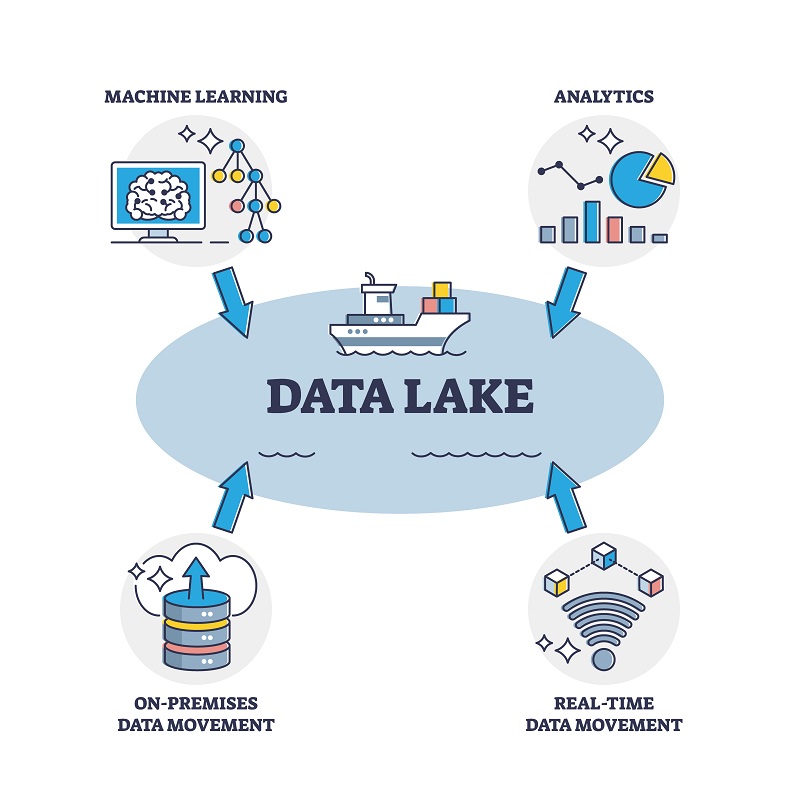
Data lake definition
A data lake is a storage repository that holds vast amounts of raw data in its native format until it's needed. Unlike a traditional data warehouse, which structures and organizes data beforehand, a data lake allows organizations to store data of all types, such as structured, semi-structured, and unstructured data, without the need for upfront transformation or schema enforcement. The concept of a data lake is closely associated with the idea of collecting and storing data without a predefined structure, enabling flexible and diverse forms of analysis.
Key features of a data lake include:
Schema Flexibility
Data lakes allow data to be ingested in its original format, whether it's structured (like databases), semi-structured (like JSON or XML), or unstructured (like text documents, images, videos). This flexibility makes it suitable for a wide range of data sources and types.
Scalability
Data lakes are designed to handle massive volumes of data, making them highly scalable. As data grows, organizations can quickly expand their storage capacity to accommodate it.
Cost Efficiency
Data lakes often use cost-effective storage solutions, such as cloud storage, which can be more affordable than traditional data warehousing solutions. This makes it feasible to store large amounts of data without incurring exorbitant costs.
Data Variety
Data lakes can store diverse types of data from various sources, enabling organizations to accumulate data from different departments, projects, and applications in a single repository.
Data Exploration
Data lakes encourage the exploration of data in its raw form. This allows data scientists and analysts to discover new insights and correlations that might need to be apparent through predefined data structures.
Data Democratization
With a data lake, access to data is less restricted. Different teams and users can access the data they need for their analyses without having to rely on IT for data preparation.
Advanced Analytics
Data lakes support advanced analytics, including machine learning, data mining, and complex queries. The ability to store both structured and unstructured data in one place facilitates these analytical processes.
Data Governance and Security
While data lakes provide flexibility, they also require robust data governance and security measures to ensure data quality, compliance, and protection against unauthorized access.
Real-time Data Processing
Some data lakes support real-time data processing, enabling organizations to analyze and react to streaming data in near-real-time.
Integration with Data Processing Tools
Data lakes can integrate with various data processing and analytics tools, allowing organizations to use their preferred tools for data preparation, transformation, and analysis.
Data Lifecycle Management
Effective data lake management involves defining data retention policies, archival strategies, and data lifecycle management to ensure that the data remains relevant and valuable over time.
Metadata Management
Metadata, which provides information about the stored data, is crucial in data lakes. Proper metadata management helps users understand and locate the data they need.
Data Lineage
Tracking the origin and transformation history of data is essential for maintaining data quality, compliance, and understanding how data has been processed.
In summary, a data lake provides a flexible and scalable environment for storing and analyzing a wide variety of data types and sources. It's particularly suitable for organizations that want to retain raw data for future analysis, enabling them to discover insights that might need to be apparent through traditional data warehousing approaches.
Running a data lake offers several advantages, but it also comes with its own set of challenges. Here are the pros and cons of operating a data lake:
Benefits of data lakes
Data lakes offer a range of benefits that make them an attractive option for organizations dealing with large and diverse datasets. One of the primary advantages of data lakes is their flexible storage capacity. They can store a wide variety of data types, including structured, semi-structured, and unstructured data, allowing organizations to maintain diverse data sources in their original format. This flexibility is crucial for businesses that need to handle complex data landscapes.
Another significant benefit of data lakes is their scalability. They are designed to manage massive amounts of data, making them highly scalable and suitable for organizations whose data volumes are continuously growing. This scalability is often coupled with cost efficiency, as data lakes typically leverage cost-effective storage solutions like cloud storage. These solutions can be more affordable than traditional on-premises options, especially for large-scale data storage needs.
In terms of data analysis and exploration, data lakes provide a robust environment. They allow data scientists and analysts to explore raw data without the need for predefining schemas. This flexibility enables the discovery of new insights and correlations that might not be apparent through traditional data warehousing approaches. Furthermore, data lakes lay the foundation for advanced analytics, including machine learning, data mining, and complex queries. They are well-suited to accommodate a wide variety of data types and sources, which is essential for comprehensive analytics.
Data lakes also contribute to data democratization within organizations. They support self-service analytics by enabling various teams and users to access the data they need without requiring IT involvement for data preparation. This accessibility fosters a more inclusive data culture within the organization.
Some data lakes are capable of real-time data processing and analysis. This feature is particularly beneficial for applications that demand quick insights from streaming data. Lastly, data lakes can seamlessly integrate with various components of the extensive data ecosystem. This integration includes compatibility with data processing frameworks like Apache Spark and analytics tools like Hadoop, further enhancing their utility in diverse data environments.
Cons of data lake
While data lakes provide significant benefits like flexibility, scalability, and support for advanced analytics, they also come with various challenges that organizations must navigate. Managing data in a data lake can become increasingly complex, especially as the volume of stored data grows. Without proper organization and metadata management, navigating and searching through the data can be difficult.
A key concern in data lakes is maintaining data quality and governance. Since data lakes store raw data without enforcing predefined structures, ensuring data quality, governance, and security becomes a challenging task. Implementing robust data governance practices is crucial to maintaining accurate and compliant data.
Another significant concern is data security. Storing a wide range of data in its raw format can increase security risks. Strong security measures are essential to protect sensitive information and prevent unauthorized access. Additionally, without appropriate organization and management, data lakes can become siloed, hindering the ability to locate and share relevant data across the organization.
Operating a data lake effectively requires skilled personnel who are versed in the technology, data integration, processing frameworks, and best practices. However, a skills gap can be a major challenge, as there might be a shortage of experienced professionals.
The variety of data types and sources in data lakes introduces complexities in data preparation, transformation, and analysis. Ingesting and preparing data for a data lake can also be time-consuming and resource-intensive, especially when dealing with diverse data sources.
Maintaining metadata is essential in making sense of the data within a data lake. Inadequate metadata management can lead to confusion and difficulty in locating relevant data. While data lakes may start with cost-effective storage solutions, the costs can accumulate over time as data volumes grow, potentially impacting the initial cost advantage.
Lastly, ensuring that teams and users understand how to effectively use a data lake and its associated tools can require significant investment in training and change management efforts.
In conclusion, data lakes offer numerous advantages but also pose challenges related to data management, security, and governance. Careful planning and implementation of a data lake strategy are essential for organizations to leverage its benefits while mitigating potential drawbacks.
Data lake examples and providers
Cloudera
Cloudera's data lake offers a range of additional benefits beyond product support, downloads, community, and documentation. As a comprehensive data management solution, it empowers organizations to unlock the full potential of their data and drive critical business intelligence (BI) insights. One of the key advantages of Cloudera's cloud data lake is its scalability and flexibility. It can handle massive volumes of structured and unstructured data, making it ideal for organizations dealing with significant data challenges.
This scalability ensures that businesses can grow their data infrastructure seamlessly as their data needs evolve. Another benefit lies in the platform's robust security features. Cloudera's data lake is designed to protect data privacy and ensure compliance with regulatory standards. Businesses can rest assured that their sensitive data is safeguarded against potential threats and unauthorized access. Moreover, the data lake facilitates advanced analytics and data processing. It offers a robust environment for data scientists and analysts to perform complex queries, run machine learning algorithms, and derive actionable insights from their data. GSK and Toyota's success stories exemplify how Cloudera's data lake has transformed their data analytics processes.
By leveraging the platform's capabilities, these organizations were able to manage and analyze their data efficiently, leading to informed decision-making and enhanced business outcomes.
Databricks
Databricks indeed stands out as a viable vendor in the data management space, offering a range of data lake alternatives that cater to diverse business needs. The Databricks Lakehouse Platform is a prime example of its innovation, combining the best elements of data lakes and warehouses to deliver a robust and comprehensive solution. With the Databricks Lakehouse Platform, businesses can leverage the reliability and scalability of data lakes, enabling them to store vast amounts of raw and unstructured data without compromising performance. At the same time, the platform incorporates the governance, security, and performance features of traditional data warehouses, ensuring data integrity and regulatory compliance.
This seamless integration of data lake and data warehouse capabilities empowers organizations to efficiently manage and analyze their data with a unified approach. Data teams can efficiently perform complex queries, advanced analytics, and machine learning tasks, deriving valuable insights to fuel their decision-making processes. The Databricks Lakehouse Platform embraces the best of both worlds, enabling businesses to break down data silos and foster collaboration across teams.
As a result, data engineers, data scientists, and analysts can work together seamlessly, accelerating the time to value and promoting a data-driven culture within the organization.
Domo
Domo, a leading data management platform, goes above and beyond to prioritize data security for its users. The platform offers advanced security features, including BYOK (bring your own key) encryption, which allows organizations to maintain complete control over their data encryption keys. This ensures an added layer of protection for sensitive data, giving businesses peace of mind when managing critical information.
Moreover, Domo provides robust control over data access and governance capabilities. Through fine-grained access controls, organizations can define who has permission to view, edit, or share specific data, enhancing data security and confidentiality. This level of governance empowers businesses to maintain compliance with industry regulations and internal data policies.
Domo Cloud, the platform's cloud-based solution, has garnered the trust of well-known corporations such as Nestle, DHL, Cisco, and Comcast. These industry giants have leveraged Domo's powerful capabilities to efficiently manage their data needs, gaining actionable insights and making informed decisions.
When should I use data lake?
A data lake pipeline is most appropriate when your organization deals with a variety of data types, including structured, semi-structured, and unstructured data, and you want to store raw data in its native format for later analysis. Here are some data lake use cases:
Data Variety:
When you have diverse data sources that include structured, semi-structured (like JSON or XML), and unstructured (like text, images, and videos) data, a data lake architecture can accommodate these various data types without the need for upfront transformations.
Exploratory Analysis:
If you're interested in exploring data without a predefined analysis path, a data lake allows data scientists and analysts to experiment with raw data and discover new insights.
Big Data Analytics:
When dealing with massive volumes of data that exceed the capacity of traditional databases or data warehouses, a data lake's scalability can handle the storage requirements.
Advanced Analytics and Machine Learning:
If your organization wants to leverage machine learning, predictive modeling, or other advanced analytics techniques, a data lake's flexibility in handling different data types is advantageous.
Real-time Data Processing:
When you need to analyze and react to streaming data in real time, a data lake can store and process these data streams effectively.
Data Exploration and Discovery:
If you're unsure about the questions you want to ask of your data and need to explore and discover insights, a data lake's flexibility allows you to store data now and analyze it later.
Data Consolidation:
When your data is dispersed across various systems, departments, and sources, a data lake can serve as a central repository for all data types, simplifying access and analysis.
Cost-Effective Storage:
If cost-efficient storage is a concern, a data lake can utilize cloud-based storage solutions, making it feasible to store vast amounts of data without excessive costs.
Data Ingestion from IoT Devices:
When dealing with data from Internet of Things (IoT) devices, which can generate a wide range of data formats, a data lake's ability to handle diverse data types is valuable.
Text and Natural Language Processing:
If you're working with text data for tasks like sentiment analysis or natural language processing, a data lake can store textual data in its raw form for analysis.
Data Enrichment:
When you want to enrich your data by combining external datasets or adding new attributes, a data lake's flexibility allows for easy integration of further information.
Data Sandbox for Innovation:
If you want to encourage innovation and experimentation by providing data scientists and analysts with a sandbox environment, a data lake supports their exploration.
In summary, use a data lake when you need to store diverse data types, support exploratory analysis, handle significant data volumes, or engage in advanced analytics and machine learning. Data lakes are well-suited for scenarios where you want to retain raw data for future analysis and discovery and where you're willing to invest in data governance and quality management processes to ensure accurate insights.
As Mobile Reality, we offer that encompass a wide range of capabilities, including predictive modeling, machine learning, data visualization, and statistical analysis. Whether it's uncovering hidden patterns, predicting future trends, or optimizing business processes, Mobile Reality's data science solutions cater to diverse industry needs.
Are you looking for trusted software agency for software developement or data science project?
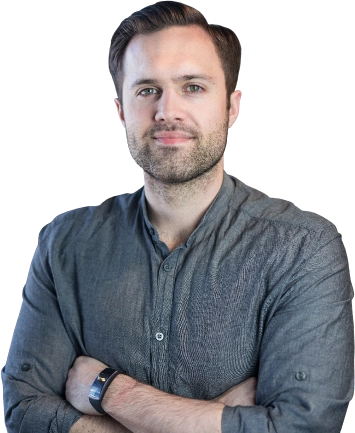
Or contact us:
Comparison of data warehouse vs data lake
Data lakes and data warehouses are both data storage and management solutions, but they differ in their approach to handling data. Here are the key differences and similarities between data lakes and data warehouses:
Difference between data warehouse and data lake
Data Structure:
Data Warehouse: Data warehouses enforce structured schemas upfront, requiring data to be transformed and loaded into a predefined format before analysis.
Data Lake: Data lakes store data in its raw, native format, allowing for flexible analysis without immediate schema enforcement.
Data Types:
Data Warehouse: Primarily designed for structured data, such as rows and columns in relational databases.
Data Lake: Can handle diverse data types, including structured, semi-structured, and unstructured data, such as text, images, logs, JSON, and more.
Processing Paradigm:
Data Warehouse: Typically used for structured data processing and analytical queries.
Data Lake: Supports batch processing, real-time streaming, and more complex and diverse data processing needs.
Schema Evolution:
Data Warehouse: Changes to the schema can be complex and require careful management due to potential impacts on downstream applications.
Data Lake: Supports schema evolution and allows for changes to the schema as data requirements evolve.
Data Transformation:
Data Warehouse: Requires extensive data transformation and cleansing before loading into the warehouse.
Data Lake: Data transformation can be deferred until analysis, allowing data scientists and analysts to shape the data as needed.
Scalability:
Data Warehouse: Scales vertically, which can become expensive as data volumes grow.
Data Lake: Scales horizontally, making it more cost-effective for storing and processing large volumes of data.
Storage:
Data Warehouse: Stores data in structured tables.
Data Lake: Stores data in files and objects, often organized in directories or folders.
Query Performance:
Data Warehouse: Optimized for structured queries and reporting.
Data Lake: Query performance depends on the data processing tools used and how data is organized.
Similarities between data warehouse and data lake
Data Integration:
Both data lakes and data warehouses can integrate data from various sources, such as operational databases, external systems, and third-party sources.
Data Governance and Security:
Both require proper data governance, security measures, access controls, and compliance management to ensure data quality, accuracy, and protection.
Analytics and Insights:
Both support analytics and insights generation by enabling data analysis, reporting, and visualization.
Business Intelligence:
Both can be used for business intelligence activities, allowing organizations to gain insights and make informed decisions based on data.
Metadata Management:
Both benefit from effective metadata management to provide context and understanding about the stored data.
Data Quality:
Both require data quality processes to ensure that the data is accurate, consistent, and reliable.
In summary, data lakes and data warehouses serve different purposes and are suited for different data scenarios. Data lakes are more flexible and versatile, handling diverse data types and allowing exploratory analysis, while data warehouses provide structured, optimized environments for structured data analysis and reporting. The choice between using a data lake or a data warehouse depends on the specific needs and characteristics of your data and analytical requirements. In some cases, organizations may even use both solutions to complement each other in their data management strategy.
Why You Don't Have to Choose
Complementary Nature: Data Warehouse and Data Lake have complementary strengths. Data Warehouses excel at providing fast and reliable access to structured data, which is crucial for generating consistent reports and conducting routine business analyses. On the other hand, Data Lakes can accommodate massive amounts of unstructured data, including text, images, and video, making them ideal for data exploration, machine learning, and other advanced analytics applications.
Scalability and Cost Efficiency
Data Lakes are highly scalable and cost-efficient when dealing with large volumes of raw data. They can accommodate both structured and unstructured data without the need for complex transformations, making them less expensive to operate and maintain. Meanwhile, Data Warehouses, with their structured storage, are more suited for quick and precise querying of smaller, processed datasets.
Flexibility in Data Processing
Data Lakes allow data scientists and analysts to experiment with various data processing and analysis techniques without worrying about data schema changes. This agility and flexibility in data processing are crucial in rapidly evolving industries and for organizations that deal with diverse and rapidly changing data types.
Integrated Data Ecosystem
Not choosing a Data warehouse vs Data Lake but integrating both Data Lakes and Data Warehouses into your data ecosystem can create a robust data management solution. By using Data Lakes as a staging area for raw data and performing the necessary data transformations, organizations can then load the processed and refined data into the Data Warehouse. This hybrid approach allows for a robust and comprehensive data platform that caters to different data needs within the organization.
Data Governance and Compliance
Data Warehouses are known for their strong data governance capabilities, providing a secure and controlled environment for sensitive data. By combining a Data Lake's flexible storage with a Data Warehouse's governance features, organizations can maintain compliance with data regulations while still leveraging the power of big data analytics.
Conclusion
In conclusion, the decision to use a data warehouse or a data lake hinges on the specific needs, goals, and characteristics of your organization's data and analytical processes. Both data warehouses and data lakes offer unique advantages and challenges, and understanding these differences is crucial for making an informed choice.
Data warehouses are ideal for structured data analysis, reporting, and historical trend analysis. They provide a structured and optimized environment for querying, making them well-suited for scenarios where data consistency, well-defined business processes, and standardized reporting are paramount. Data warehouses excel in methods requiring complex queries, where the data is well-structured and originates from various operational sources. However, they do need careful data transformation and schema design upfront, as well as investment in data governance and security measures.
On the other hand, data lakes shine when dealing with diverse data types and sources, including structured, semi-structured, and unstructured data. They support exploratory analysis, advanced analytics, and machine learning, making them suitable for scenarios where the data landscape is rapidly evolving or where the analytical path is not predefined. Data lakes offer the flexibility to store raw data in its native format, allowing data scientists and analysts to derive insights later. However, they require robust metadata management and data governance to ensure accurate analysis and proper security.
While data lakes and data warehouses have distinct purposes, they share similarities in terms of data integration, data governance, analytics capabilities, and the need for high data quality. Organizations often find themselves evaluating these solutions based on their data landscape, analytical goals, and budget constraints. In some cases, a hybrid approach that integrates both solutions might offer the best of both worlds.
Ultimately, the key is to assess your organization's data needs, consider the types of data you work with, and align your decision with your analytical goals and business strategies. By carefully considering the pros, cons, features, and best-fit scenarios for both data lakes and data warehouses, you can make a strategic choice that empowers your data-driven initiatives and supports the growth of your organization.
Did you like the article?Find out how we can help you.
Matt Sadowski
CEO of Mobile Reality
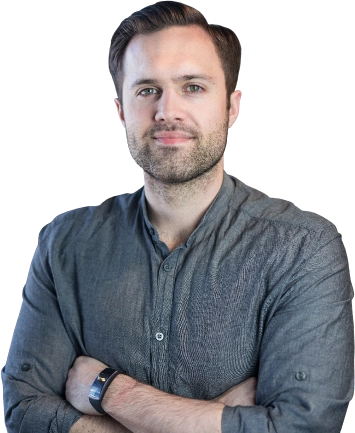
Related articles
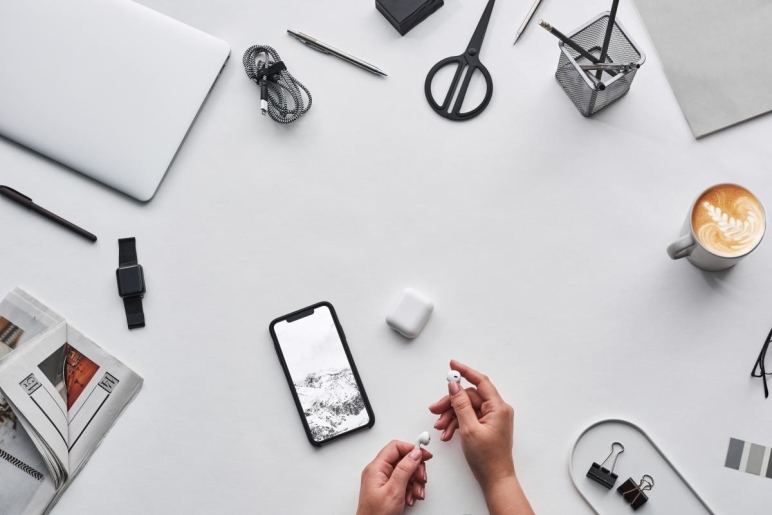
26.04.2024
TOP 6 Myths of Business Intelligence Debunked
Business analytics (or Business Intelligence, BI) is less talked about today than Big Data or artificial intelligence.
Read full article
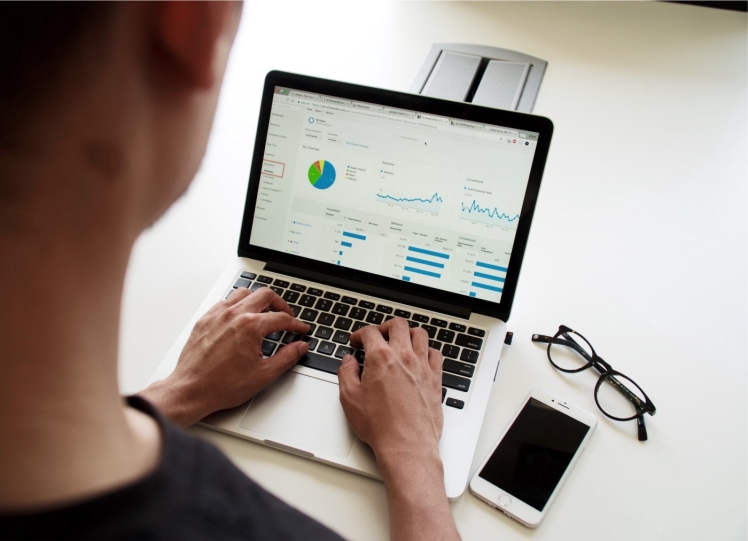
26.04.2024
Blocks of Modernity in Analytics
The exponential growth of digital technology, ubiquitous internet connectivity, and proliferation of smart devices.
Read full article
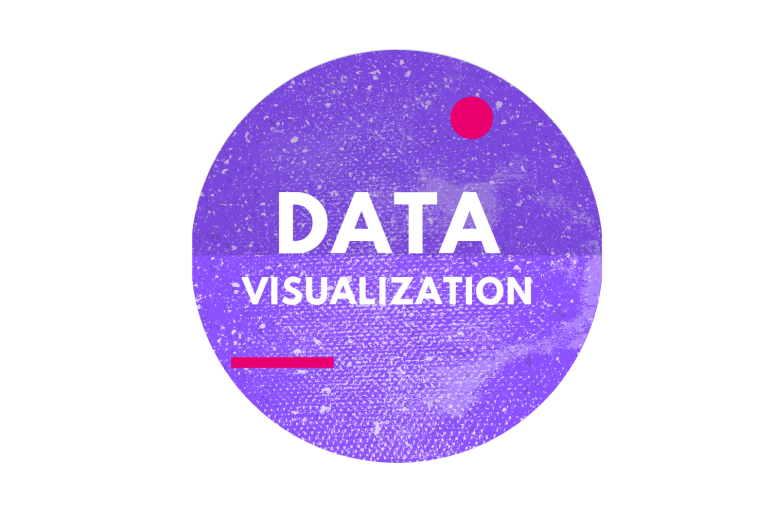
26.04.2024
Advantages and Disadvantages of Tableau
Tableau Software as a data visualization tool for non-dev users. In this article, we show you its' advantages and disadvantages.
Read full article